Data Warehouse vs. Data Lake vs. Data Mesh – Finding your way through data architectures
November 23, 2022
As more and more organizations are driving informed decision-making by leveraging data, the reliance on data management infrastructure has become imperative for a seamless customer experience.
Experts at Domo found that in 2017, 2.5 quintillion bytes of data were generated every day of the year, expecting the figures to increase up to 463 exabytes in 2025. However, unprecedented events like COVID-19 have already disrupted data generation sequences earlier than expected.
Therefore, data modernization has become imperative for businesses to stay ahead of the curve. Data warehouse, data lake, and data mesh are developed for successful big data management, better business intelligence, reliable ML workloads, and robust analytics. This article defines core frameworks or platforms that helped our clients effectively leverage data for business & cost optimization and truly become data-driven organizations.
What is a Data Warehouse?
The data warehouse was the first platform for effective data utilization to support business. The traditional data warehouse supports heterogeneous data sources, categorizes them, and stores the data for future use. Users can simultaneously utilize data along with high performance. A traditional data warehouse can further be classified into 3 further divisions:
1- Single-tier data warehouse architecture
2- Two-tier data warehouse architecture
3- Three-tier data warehouse architecture
Deep diving into the data warehouse architecture
Commonly, the data warehouse architecture includes a staging area for all data sources before the data is moved into warehouse layers. The staging layer ensures that data is properly cleansed and available in an appropriate format. Processing is in the next layer, whereas the final records are presented at the end. A data warehouse records data in an ACID-compliant manner to ensure the highest levels of integrity.
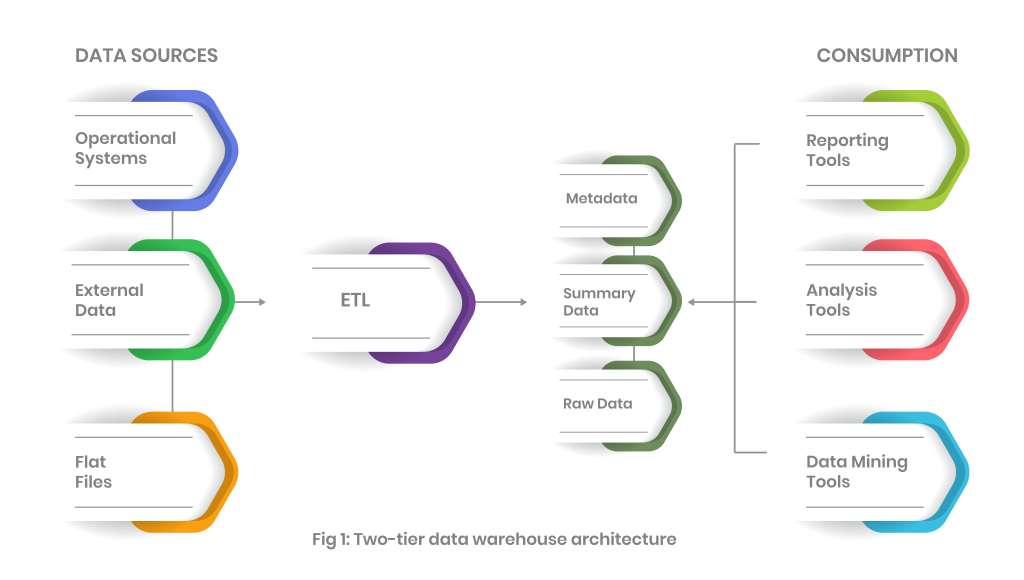
Typically, a data warehouse combines relational data sets from numerous sources, cleansing and loading this into the warehouse systems, where it serves as a single source of data truth. By using traditional data warehouses, data engineers, business analysts, and data scientists can take strategic decisions via BI tools, SQL clients, and analytics applications.
The case ‘for’ and ‘against’ data warehouse
Pros | Cons |
Faster data access | Time-consuming preparation |
Speedy analytics and BI workloads | Data ownership concerns |
Error identification & correction | Scarcity of unique data |
Data quality and consistency | Data homogenization |
Reduce response times | Data processing limitations |
What is a data lake?
Next to the data warehouse, a data lake offers more advanced, centralized, and flexible storage options that can ingest large data in structured/unstructured form. A data lake on the other hand, when compared to a traditional data warehouse, uses a flat data architecture with raw-form object storage.
Data lakes are more advanced in terms of flexibility, durability, and cost optimization. Data lakes enable the organization to gain more advanced insight. Data lakes also support machine learning and predictive analytics by utilizing data generated from multiple sources like IoT devices, social media, and streaming data.
Understand the data lake architecture
When it comes to a data lake, the data, interchangeably called the schema, is not defined. Alternatively, it is extracted and converted to use in the analysis stage.
Data lake – The risks and rewards
A data lake enables the organization to manage large data sets more effectively because they offer seamless access to underlying data. Organizations can have a unified view, irrespective of storage type.
Pros | Cons |
Support diverse workloads | Deployment complexity |
Governance/discovery/search | Data quality issues |
Lesser data duplications | Security |
Support diverse data types | Metadata life cycle management |
Processing/storage decoupling | Integration complexity |
Lower TOC | Compute cost management |
Acid transaction support | |
Schema enforcement | |
End-to-end streaming |
Related concept: What is the lake house?
A data lake house is a relatively new, open data management architecture. It comes with the coupled flexibility, cost optimizations, and scale of a modern data lake while adding value to the data management and ACID transaction capacity of the data warehouse. This concept enables more advanced business intelligence (BI) and support for machine learning on all data.
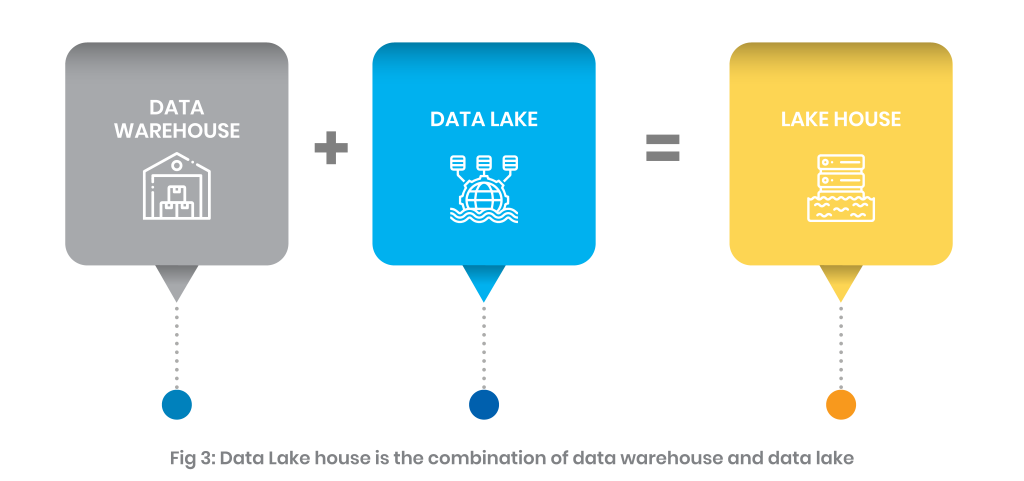
A data Lakehouse addresses the key problems that arise with the traditional two-tier architecture of data warehouses and data lakes. This includes reliability, lower TOC, data staleness, and advanced analytics at unified storage. We’ve already explained the details of a modern-day connected lake house in our previous article.
Salient features of a lake house
- Real-time data management
- Schema support
- A mechanism for data governance
- Direct access to the data sources
- End-to-end steaming
- Support for disparate data sources
- Lesser data redundancy and movement
What is data mesh?
Simply put, a data mesh is a data architecture for managing your data through a decentralized approach. It allows your end-users including customers, suppliers, employees and stakeholders to readily access important data without having to migrate it to other data sources. The term, first coined by Zhamak Dehghani presents data mesh as a rather sociotechnical way of data acquisition, management, and sharing based on cutting-edge analytical requirements.
In a data mesh, you basically break down a monolithic data management platform into manageable components using a decentralized approach that distributes data and allows domain-specific teams to take ownership while serving data as a product only.
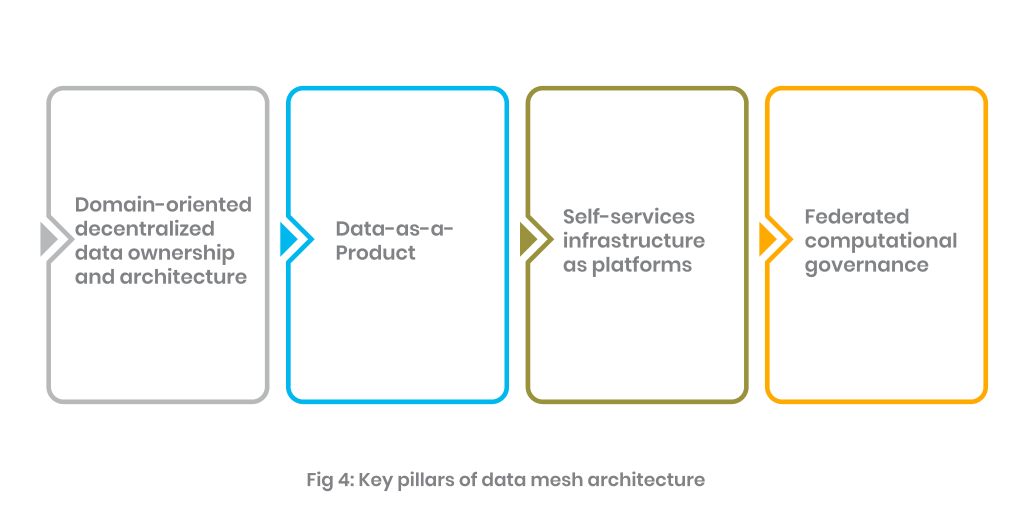
Data mesh address three major issues that users were facing in previous architecture
1- Data is not easily accessible by data consumers.
2- Too much back and forth between data consumers (i.e., data sciences teams, domain users, consumption layer owners)
3- Lower the burdens of central data teams tasked with being the purveyors of data.
The idea revolves around the fact that domain teams can control their data and can support projects where data is required by minimizing the intervention of data scientists. Follow our detailed article on data mesh, its working and key principles here!
Analyzing the data mesh architecture
A data mesh architecture comprises three components: data sources, data infrastructure, and domain-oriented data pipelines, all of which are managed by functional owners. Underneath the entire architecture is a layer of global interoperability with domain-agnostic protocols, as well as reliability and accountability
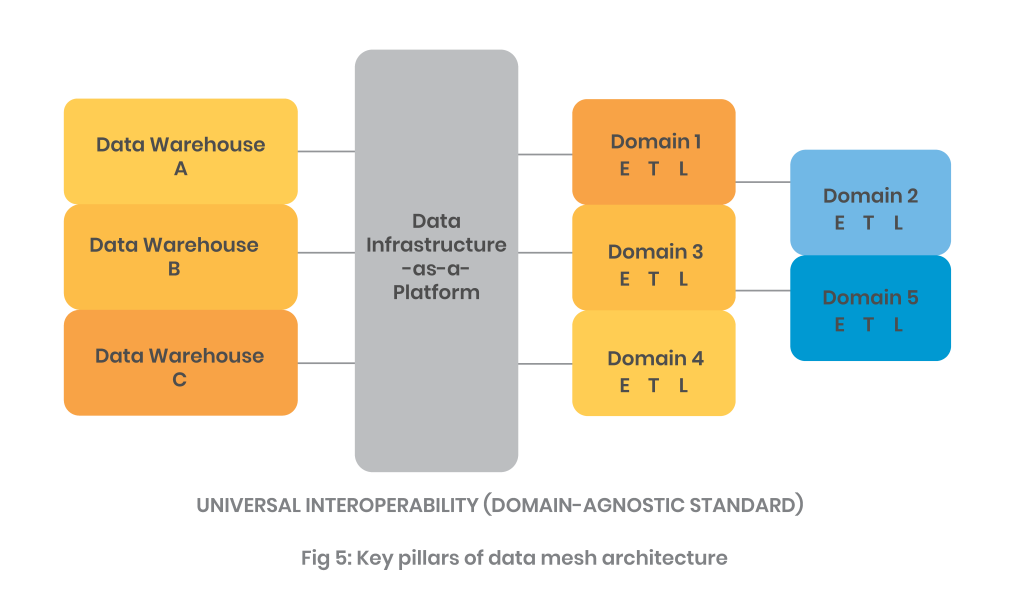
Data mesh: Advantages vs disadvantages
Pros | Cons |
Agility & scalability | Failure to automatically update data catalogues |
Flexibility and Independence | Excessive Involvement of domain teams |
Faster access to data | Shadow data analytics and mandates |
Cross-functional Use | Failure to accurately evolve data products |
Data transparency | Integration complexity |
Data warehouse, data lake, or data mesh: Which one is chosen?
A data warehouse is a solid option for organizations looking for a mature data landscape with structured data solutions that focus on dependable analytics. Whereas, data lakes are suitable for organizations seeking more flexibility with a lower cost of ownership, big data solutions, and support for machine learning, predictive analytics, and data science workload on structured or unstructured data.
Data Mesh, a recently introduced concept, solves data processing bottlenecks with complex yet powerful systems. With its powerful data processing function, the enterprise data mesh is becoming a foundational enabler of real-time digital enterprises.
Quick Link
You may like
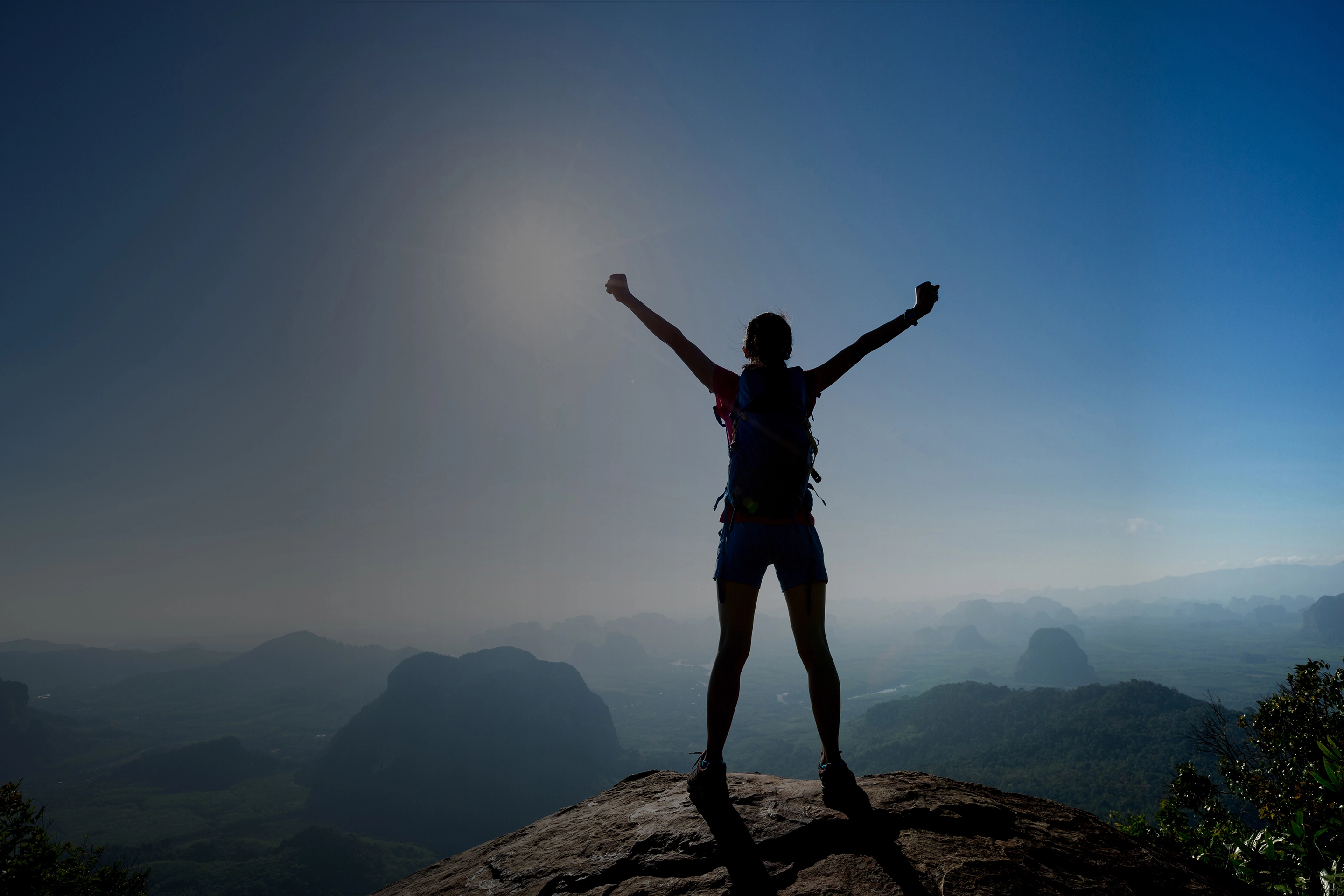
From data to decisions: Exploring data analytics in banking
Learn more about how digital currency is the new talk of the digital age, revolutionizing the way
READ MOREHow can we help you?
Are you ready to push boundaries and explore new frontiers of innovation?